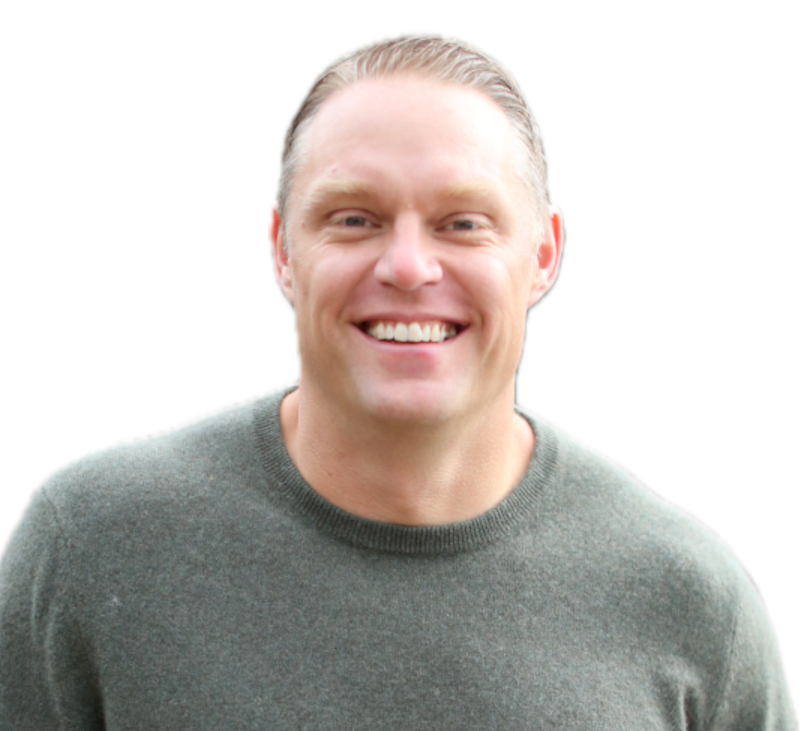
Mainframe workloads are costly, rigid, and difficult to scale. As enterprises look for modernization strategies, IaC with Terraform provides a seamless way to transition these legacy systems into scalable Kubernetes (EKS) environments. This blog explores how enterprises can leverage Terraform to provision, manage, and scale cloud-based infrastructure that supports modernized COBOL workloads while reducing operational complexity.
The Need for Modernization
Many organizations rely on COBOL-based batch processing that runs on mainframes. These systems are:
- Expensive: High licensing and maintenance costs.
- Inflexible: Limited scalability for growing workloads.
- Resource-Intensive: Requires specialized skills that are increasingly rare.
Solution: Automating AWS Infrastructure with Terraform
By defining cloud resources as code, Terraform enables organizations to:
Deploy and manage AWS EKS clusters efficiently.
Automate provisioning of EFS storage for legacy data.
Implement IAM roles with least-privilege policies for security.
Maintain version-controlled infrastructure using GitOps.
Infrastructure Overview
This solution deploys an AWS-backed Kubernetes environment where COBOL-based data processing runs as a scheduled Kubernetes CronJob. The following diagram illustrates the architecture:
- EKS Cluster: Hosts containerized COBOL applications.
- EFS Storage: Stores processed CSV data.
- IAM Roles & Policies: Provides secure access control.
- Terraform Automation: Manages cloud resources as code.
Step-by-Step Implementation with Terraform
1. Deploying an AWS EKS Cluster
The following Terraform script provisions an Amazon EKS cluster with worker nodes.
module "eks" {
source = "terraform-aws-modules/eks/aws"
version = "20.31.6"
cluster_name = local.environment
authentication_mode = "API_AND_CONFIG_MAP"
cluster_version = var.eks_cluster_version
cluster_endpoint_private_access = true
cluster_endpoint_public_access = true
cluster_ip_family = "ipv4"
cluster_addons = {
aws-ebs-csi-driver = {
service_account_role_arn = module.ebs_csi_irsa_role.iam_role_arn
most_recent = true
}
aws-efs-csi-driver = {
service_account_role_arn = module.efs_csi_irsa_role.iam_role_arn
most_recent = true
}
coredns = {
most_recent = true
}
kube-proxy = {
most_recent = true
}
vpc-cni = {
most_recent = true
before_compute = true
configuration_values = jsonencode({
env = {
ENABLE_PREFIX_DELEGATION = "true"
WARM_PREFIX_TARGET = "1"
}
})
}
}
iam_role_additional_policies = {
AmazonEC2ContainerRegistryReadOnly = "arn:aws:iam::aws:policy/AmazonEC2ContainerRegistryReadOnly"
}
enable_cluster_creator_admin_permissions = true
cluster_tags = var.tags
vpc_id = module.vpc.vpc_id
subnet_ids = module.vpc.private_subnets
eks_managed_node_groups = {
gpu_node_group = {
ami_type = "AL2_x86_64_GPU"
instance_types = [var.eks_node_gpu_instance_type]
min_size = 1
max_size = 3
desired_size = 1
use_latest_ami_release_version = true
ebs_optimized = true
enable_monitoring = true
block_device_mappings = {
xvda = {
device_name = "/dev/xvda"
ebs = {
volume_size = 75
volume_type = "gp3"
encrypted = true
delete_on_termination = true
}
}
}
labels = {
gpu = true
"nvidia.com/gpu.present" = true
}
pre_bootstrap_user_data = <<-EOT
#!/bin/bash
set -ex
# Install dependencies
yum install -y cuda
# Add the NVIDIA package repositories
distribution=$(. /etc/os-release;echo $ID$VERSION_ID)
curl -s -L https://nvidia.github.io/nvidia-docker/$distribution/nvidia-docker.repo | sudo tee /etc/yum.repos.d/nvidia-docker.repo
# Install the NVIDIA container runtime
sudo yum install -y nvidia-container-toolkit
EOT
}
node_group = {
ami_type = "AL2_x86_64"
instance_types = [var.eks_node_instance_type]
min_size = 1
max_size = 5
desired_size = 1
use_latest_ami_release_version = true
ebs_optimized = true
enable_monitoring = true
block_device_mappings = {
xvda = {
device_name = "/dev/xvda"
ebs = {
volume_size = 75
volume_type = "gp3"
encrypted = true
delete_on_termination = true
}
}
}
labels = {
gpu = false
}
iam_role_additional_policies = {
AmazonSSMManagedInstanceCore = data.aws_iam_policy.AmazonSSMManagedInstanceCore.arn
eks_efs = aws_iam_policy.eks_efs.arn
}
tags = var.tags
}
}
}
2. Setting Up EFS for Persistent Data Storage
Legacy workloads often require shared storage for processing large CSV files. AWS EFS provides scalable, persistent storage.
module "efs" {
source = "terraform-aws-modules/efs/aws"
version = "1.6.5"
name = var.environment
creation_token = var.environment
encrypted = true
mount_targets = { for k, v in zipmap(local.availability_zones, module.vpc.private_subnets) : k => { subnet_id = v } }
security_group_description = "${var.environment} EFS security group"
security_group_vpc_id = module.vpc.vpc_id
security_group_rules = {
vpc = {
description = "Ingress from VPC private subnets"
cidr_blocks = module.vpc.private_subnets_cidr_blocks
}
}
tags = var.tags
}
Terraform Workflow for AWS Infrastructure
- Define Infrastructure in Terraform (
.tf
files). - Initialize Terraform to download AWS provider plugins.
- Plan Deployment to preview changes.
- Apply Changes to provision AWS resources.
- Monitor & Manage infrastructure via Terraform state files.
.
├── backend.tf
├── ecr.tf
├── efs.tf
├── eks.tf
├── k8s.tf
├── local.tf
├── outputs.tf
├── plan.out
├── terraform.tfvars
├── variables.tf
├── versions.tf
└── vpc.tf
Deploying the Infrastructure
1. Initialize Terraform
terraform init
2. Plan Infrastructure Deployment
terraform plan -out=infra_plan.out
3. Apply Changes to Provision AWS Resources
terraform apply infra_plan.out
Once applied, Terraform provisions:
- An EKS Cluster.
- EFS Storage for data persistence.
- IAM roles and policies for security.
Scaling & Automation Benefits
Using Terraform for AWS infrastructure automation provides several advantages:
Scalability: Easily scale Kubernetes workloads across multiple nodes.
Cost Savings: Optimized resource provisioning reduces cloud costs.
Security: Enforced IAM policies and secure storage solutions.
Agility: Version-controlled infrastructure allows easy rollbacks and updates.
Final Thoughts
By leveraging Terraform to automate the deployment of AWS-based Kubernetes environments, enterprises can modernize mainframe workloads, reduce costs, and enhance scalability—all while maintaining security and operational control.